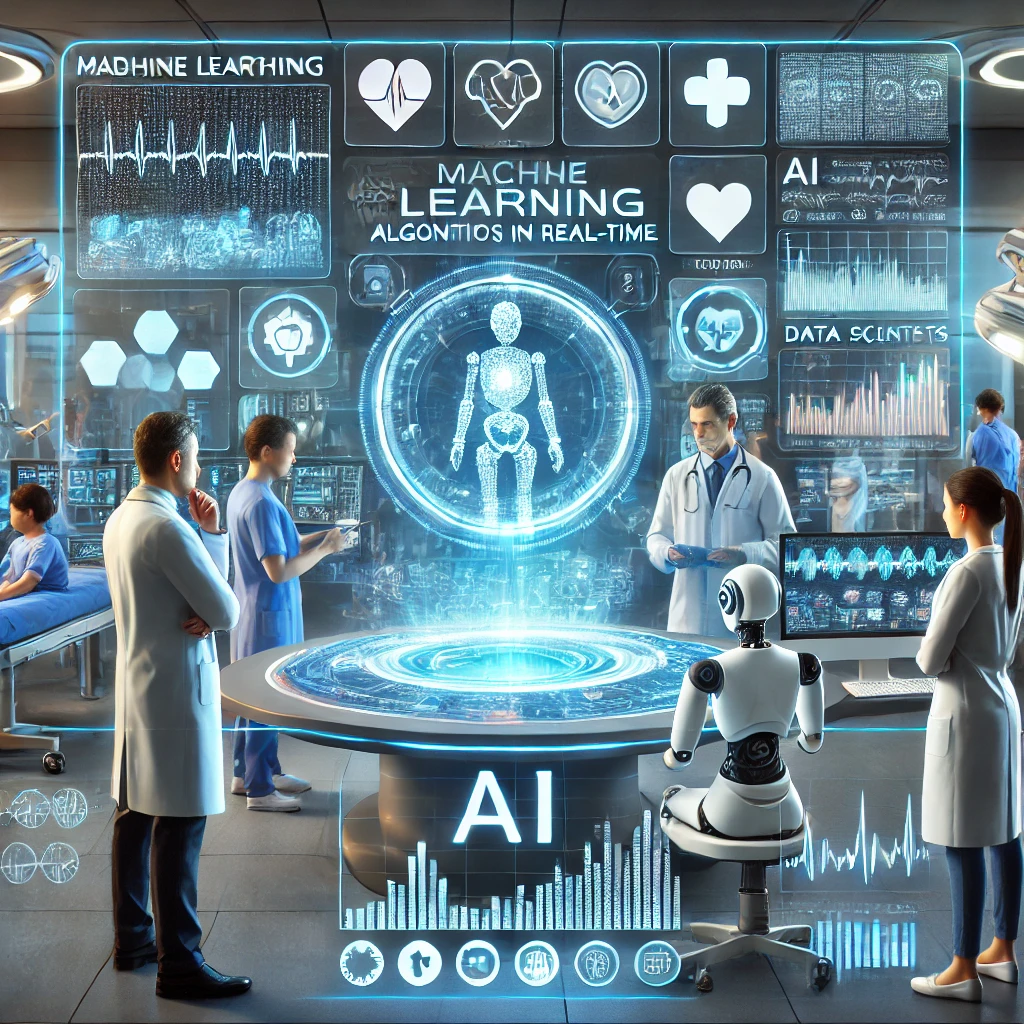
The healthcare industry has witnessed remarkable advances in the last few decades, with technology playing a pivotal role in this transformation.
Among these technological innovations, data science stands out as a driving force in revolutionizing how healthcare operates.
By extracting meaningful insights from vast amounts of medical data, data science is not only improving patient care but also streamlining operational efficiency and reducing costs.
From predictive analytics to personalized treatments, data science is reshaping healthcare for the better.
In this article, we’ll explore the key ways in which data science is revolutionizing healthcare, including some fascinating real-world examples.
Additionally, I will guide you to my other blog post that delves into how artificial intelligence (AI) is complementing data science to further revolutionize healthcare.
Predictive Analytics for Early Diagnosis and Prevention
One of the most powerful applications of data science in healthcare is predictive analytics.
Using historical patient data, predictive analytics algorithms can help identify the likelihood of future health issues before they manifest.
Early diagnosis can be critical for diseases like cancer, heart disease, and diabetes, where timely intervention significantly improves outcomes.
For example, hospitals are now using machine learning models to predict patient deterioration in Intensive Care Units (ICUs).
These models analyze data points like heart rate, blood pressure, oxygen levels, and past medical history to predict the likelihood of a patient’s condition worsening. This allows healthcare professionals to intervene sooner, potentially saving lives.
Additionally, predictive analytics is being applied to population health management.
By analyzing data from large groups of patients, healthcare providers can identify at-risk populations and implement preventive measures, such as recommending lifestyle changes or early screening programs.
In the case of diabetes, for instance, predictive models can assess risk factors such as age, weight, family history, and lifestyle choices to predict who might develop the disease, allowing for early intervention.
Personalized Treatment Plans Tailored to Individual Patients
Gone are the days of the “one-size-fits-all” treatment approach. Thanks to data science, personalized medicine is becoming a reality.
Personalized medicine, also known as precision medicine, uses data to tailor treatments based on an individual’s genetic makeup, lifestyle, and medical history. This leads to more effective treatments and fewer side effects.
One prominent example of personalized treatment is in oncology, where genomic data is being used to develop cancer therapies specifically designed for individual patients.
By analyzing a patient’s genetic mutations, oncologists can select treatments that target those specific mutations, making therapies more effective. This approach has already shown success in treating cancers like melanoma and breast cancer.
Beyond cancer, personalized treatment is making strides in managing chronic diseases.
For example, data science tools can analyze the behavior patterns of patients with diabetes or cardiovascular diseases to adjust medication dosages and recommend lifestyle changes in real-time.
Wearable devices, such as glucose monitors, are generating continuous data streams that can be analyzed by machine learning algorithms to offer more personalized care.
Enhancing Clinical Decision-Making with Data-Driven Insights
Data science tools are also assisting doctors in clinical decision-making by providing evidence-based insights.
With the sheer volume of medical research available today, it’s nearly impossible for physicians to keep up with every new study or breakthrough.
Data science algorithms can analyze this vast corpus of medical knowledge and provide healthcare professionals with the most relevant, up-to-date information for making decisions.
For example, IBM’s Watson for Health uses natural language processing (NLP) to scan thousands of medical journals, research papers, and clinical trials to assist doctors in diagnosing diseases and recommending treatments.
By providing real-time data, Watson helps physicians offer more accurate diagnoses and select the most effective treatment options.
Clinical decision support systems (CDSS) are also leveraging data science to minimize human errors in prescribing medication, particularly for patients with complex medical histories.
These systems alert doctors when potential drug interactions or allergic reactions might occur, ensuring that treatments are as safe as possible.
Revolutionizing Drug Discovery and Development
The process of discovering and developing new drugs has historically been long and expensive. On average, it takes over a decade and billions of dollars to bring a new drug to market.
However, data science is accelerating the drug development process by helping pharmaceutical companies analyze large datasets to identify potential drug candidates faster and more efficiently.
By using machine learning algorithms, researchers can sift through massive datasets, such as genomic data and clinical trial results, to identify patterns and potential drug targets.
For example, companies like Atomwise and BenevolentAI are using deep learning algorithms to predict how different molecules will interact with biological targets, drastically speeding up the drug discovery process.
Additionally, data science is improving clinical trials by identifying the right patients for trials based on their genetic profiles and medical histories.
This not only increases the chances of a successful trial but also reduces costs by ensuring that trials are conducted on patients most likely to benefit from the treatment.
Reducing Hospital Readmissions with Predictive Models
Hospital readmissions are a significant challenge for healthcare providers. High readmission rates are costly for hospitals and can indicate poor quality of care.
However, data science is helping reduce readmissions by using predictive models to identify patients at risk of being readmitted after discharge.
By analyzing factors like previous admissions, lab results, and socioeconomic conditions, predictive models can flag patients who are likely to need additional care after leaving the hospital.
These insights enable healthcare providers to implement follow-up plans, such as home visits or telemedicine consultations, to ensure patients receive the care they need post-discharge.
This proactive approach not only improves patient outcomes but also reduces the financial burden on healthcare systems by minimizing the need for readmissions.
Optimizing Hospital Operations and Resource Allocation
Data science is also being used to improve the operational efficiency of hospitals.
From managing staff schedules to optimizing the use of medical equipment, data-driven tools can streamline hospital operations, reducing costs and improving patient care.
For example, predictive models can forecast patient admission rates, helping hospitals allocate resources more effectively. Hospitals can better manage staffing levels, ensuring they have enough doctors and nurses to handle patient inflows without being overstaffed during quieter periods.
Similarly, operating rooms and medical equipment can be scheduled more efficiently to reduce wait times and avoid bottlenecks.
Data science is also being applied to reduce waste in hospitals.
For example, some hospitals are using machine learning to predict the amount of medical supplies they’ll need, minimizing the risk of overstocking or understocking essential materials.
Advancing Mental Health Care with Data Science
Data science is playing an increasingly important role in improving mental health care. Mental health conditions like depression and anxiety are often underdiagnosed or misdiagnosed due to the subjective nature of diagnosis.
However, machine learning models can help identify patterns in patient data that signal mental health conditions, improving diagnostic accuracy.
For example, researchers are using natural language processing to analyze the tone and sentiment of patients’ written or spoken language.
Changes in language patterns can be early indicators of depression or other mental health conditions, allowing for early intervention.
Additionally, wearable devices that track physiological data like heart rate and sleep patterns can provide insights into a patient’s mental health.
By analyzing this data, machine learning models can alert healthcare providers when a patient’s mental health is deteriorating, allowing for timely interventions.
Telemedicine and Remote Monitoring
Telemedicine has grown exponentially in recent years, particularly in response to the COVID-19 pandemic. Data science plays a crucial role in enabling telemedicine by providing real-time insights into a patient’s health.
Remote monitoring tools, such as wearable devices and connected health apps, allow doctors to track patients’ health without needing in-person visits.
For example, patients with chronic conditions like diabetes or hypertension can use wearable devices that continuously monitor their vital signs. These devices send data to healthcare providers in real-time, allowing doctors to adjust treatment plans based on the latest information.
Machine learning algorithms can analyze this data to detect anomalies or trends that require attention.
Telemedicine and remote monitoring are especially valuable in rural or underserved areas, where access to healthcare facilities may be limited.
Data-driven insights enable patients to receive high-quality care from the comfort of their homes, improving access to healthcare while reducing costs.
Fighting Pandemics with Data
Data science has proven to be a powerful tool in fighting pandemics, most notably during the COVID-19 crisis.
Predictive models helped healthcare providers and governments track the spread of the virus, allocate resources effectively, and make data-driven public health decisions.
For instance, epidemiological models used data from infection rates, travel patterns, and population density to predict where outbreaks were likely to occur next.
These models enabled authorities to implement targeted lockdowns and allocate medical supplies to areas with the greatest need.
Data science also played a role in vaccine development. Researchers used machine learning models to analyze massive amounts of data from clinical trials, speeding up the process of testing vaccine efficacy and safety.
Reducing Healthcare Costs with Data Science
As healthcare systems face rising costs, data science offers a way to reduce expenses while maintaining or even improving the quality of care.
Predictive analytics can help healthcare providers identify unnecessary tests, treatments, or hospitalizations, streamlining care and reducing costs.
For example, some hospitals are using predictive models to identify patients who are unlikely to benefit from certain procedures or medications.
By avoiding unnecessary interventions, healthcare providers can reduce costs and improve patient outcomes.
Data science is also enabling more efficient use of healthcare resources, such as operating rooms, medical equipment, and staff, further reducing operational costs.
Learn More: How AI is Complementing Data Science in Healthcare
While data science is revolutionizing healthcare, artificial intelligence (AI) is playing a complementary role. AI is enhancing the power of data science by automating processes, improving accuracy, and speeding up decision-making.
To learn more about how AI is revolutionizing healthcare, check out my blog post on How AI is Revolutionizing Healthcare on my website.
In that post, I delve deeper into AI’s role in areas like drug discovery, predictive healthcare, and personalized treatment.
Conclusion
Data science is transforming every aspect of healthcare, from patient diagnosis to hospital operations.
By leveraging data-driven insights, healthcare providers can offer more personalized, efficient, and cost-effective care.
As the field of data science continues to evolve, we can expect even more innovations that will shape the future of healthcare.
For more insights into how data science and AI are revolutionizing healthcare, make sure to explore the other blogs on my website!